Ahmad Faraz Khan
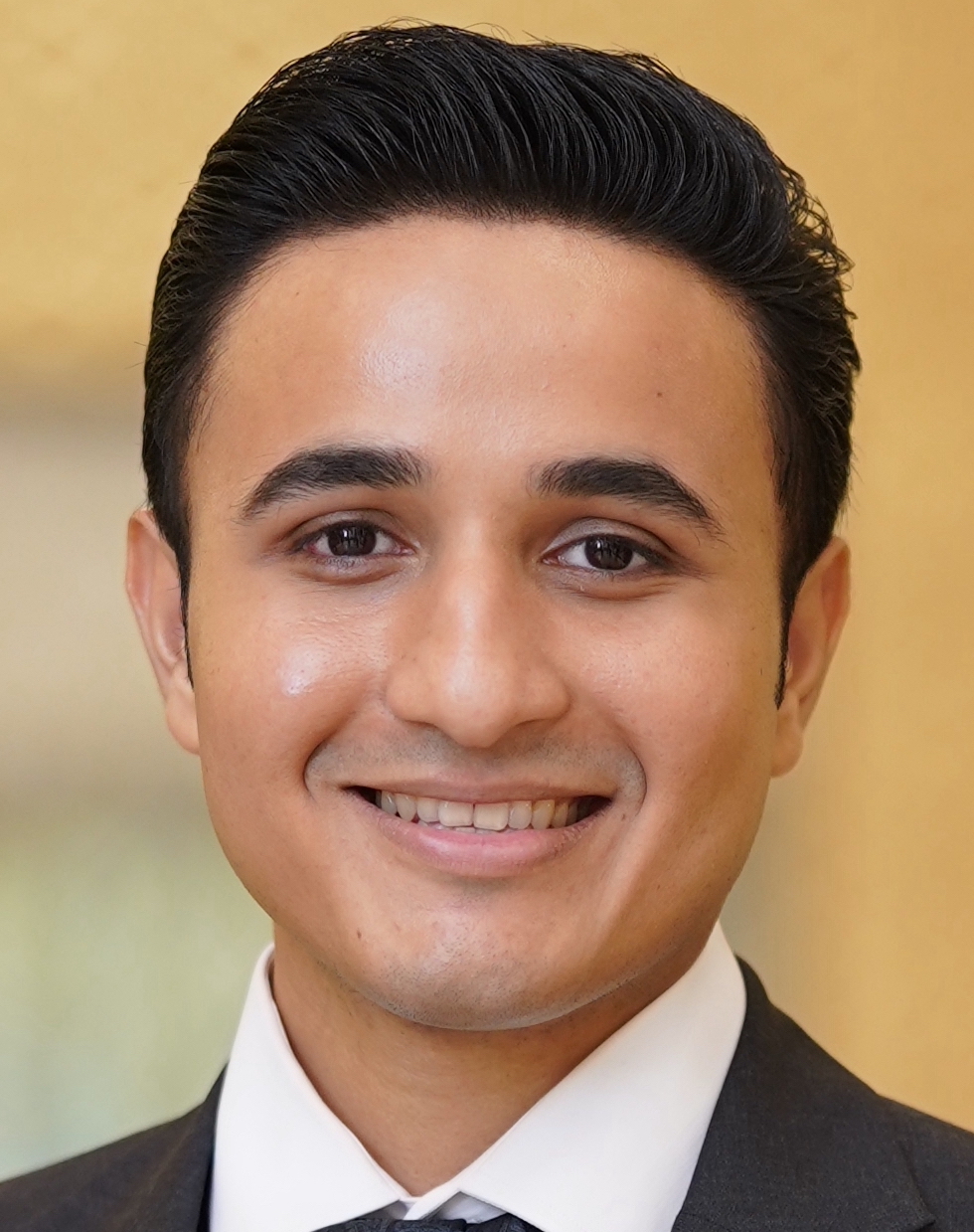
I am a fifth-year Ph.D. candidate in Computer Science at Virginia Tech in the DSSL lab working with Dr. Ali R. Butt. My research is focused on Machine Learning Systems and Federated Learning.
Currently, I am working on (1) Sys4ML: Enhancing resource utilization, scalability, and efficiency of distributed learning on resource-constrained systems by developing specialized (computing and storage) systems for distributed ML. (2) ML4Sys: (i) Personalized ML: Developing enhanced personalized learning solutions for distributed ML systems. (ii) Incentivized ML: Improving incentive mechanisms within resource-constrained distributed ML systems to ensure fairness and adaptability. (3) LLMs fine-tuning and optimization: Fine-tuning LLMs to reduce sycophancy and prompt optimization for specific tasks. (4) Privacy-aware LLMs: Fine-tuning LLMs with privacy-aware data and utilizing LLMs as human replacements for human in the loop Federated Learning.
I did my B.S. in Computer Science from LUMS University working with Dr. Ihsan Ayyub Qazi and Dr. Zafar Ayyub Qazi.
news
Jul 31, 2025 |
![]() ![]() ![]() ![]() ![]() |
---|---|
Jun 03, 2025 |
![]() ![]() ![]() ![]() ![]() ![]() ![]() |
Dec 16, 2024 |
![]() ![]() |
Dec 15, 2024 |
![]() ![]() ![]() ![]() ![]() |
Feb 24, 2024 | Serving on the external review committee for ATC 2024. |
selected publications
-
FLStore: Efficient Federated Learning Storage for non-training workloadsIn Eighth Conference on Machine Learning and Systems (MLSys ’25) , 2025
-
IP-FL: Incentive-driven Personalization in Federated LearningIn 39th IEEE International Parallel & Distributed Processing Symposium (IPDPS ’25) , 2025
-
FLOAT: Federated Learning Optimizations with Automated TuningIn Nineteenth European Conference on Computer Systems (EuroSys ’24) , 2024
-
Mitigating Sycophancy in Large Language Models via Direct Preference OptimizationIn 2024 IEEE International Conference on Big Data (BigData) , Dec 2024
-
DynamicFL: Federated Learning with Dynamic Communication Resource AllocationIn 2024 IEEE International Conference on Big Data (BigData) *Awarded Best Paper* , Dec 2024